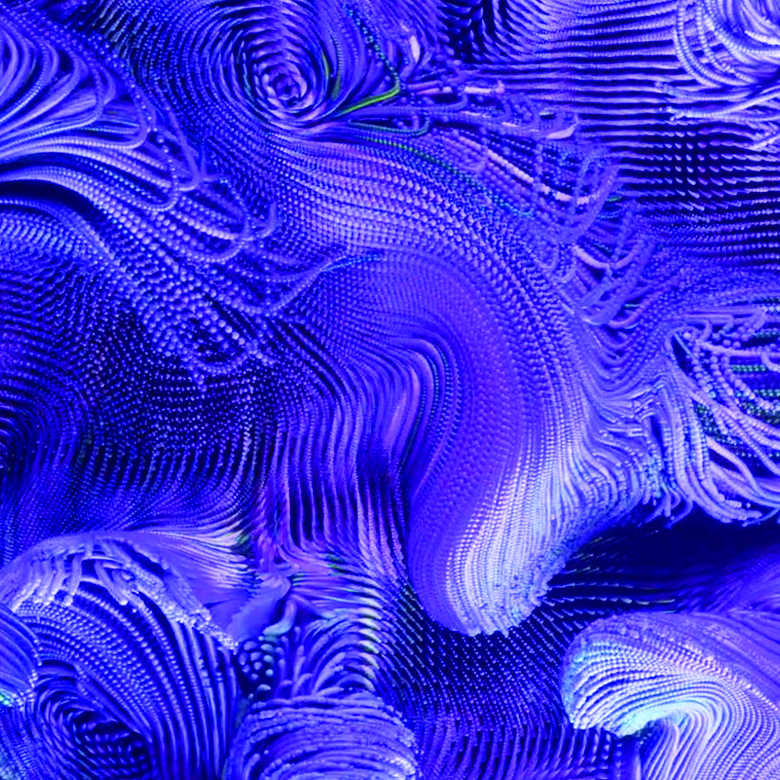
Future Faster
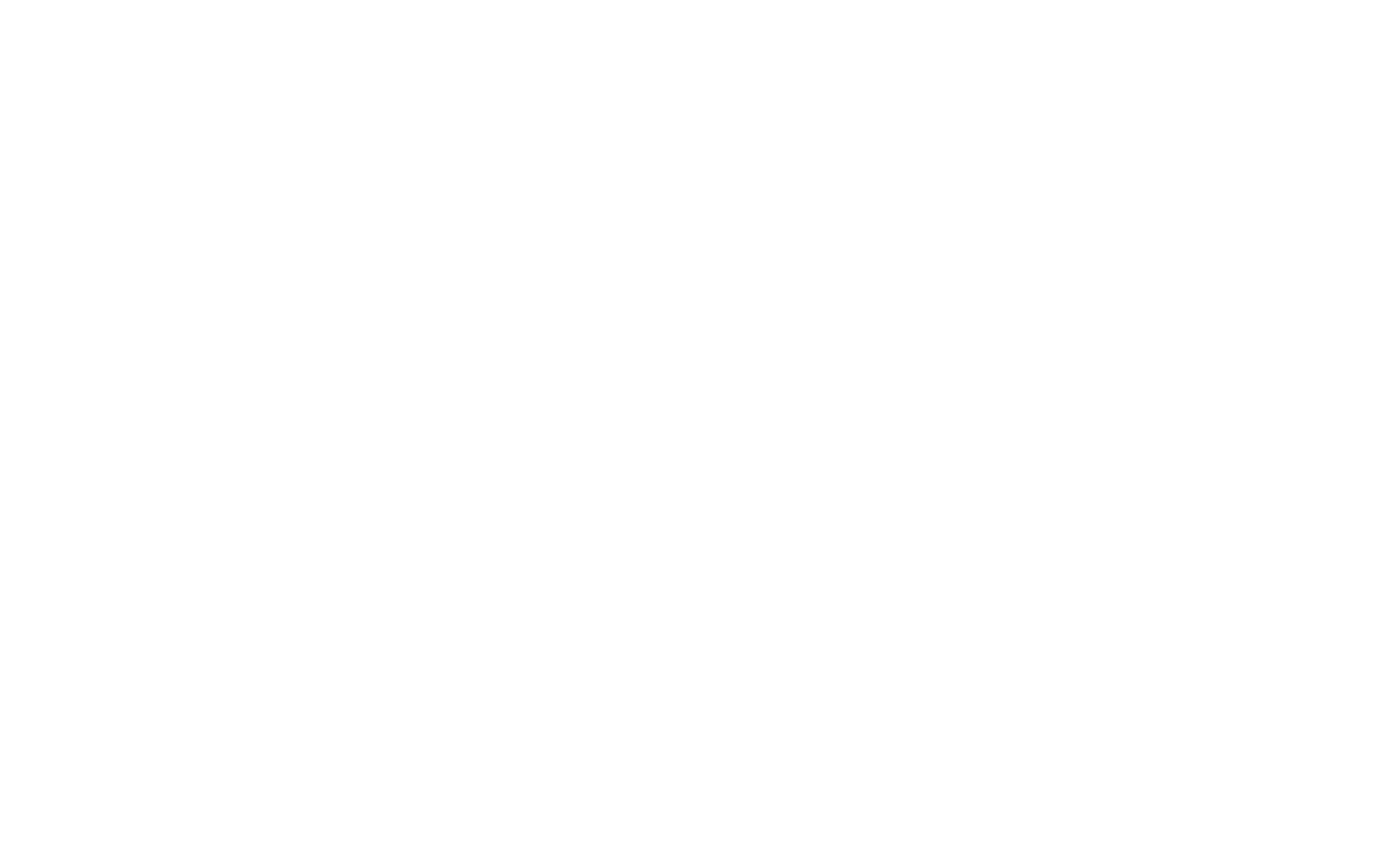
Our core beliefs
Our core beliefs
Everything is information
Change isn’t meant to be feared
Quantity is quality
Talent is global, opportunity is not
Everything is
information.
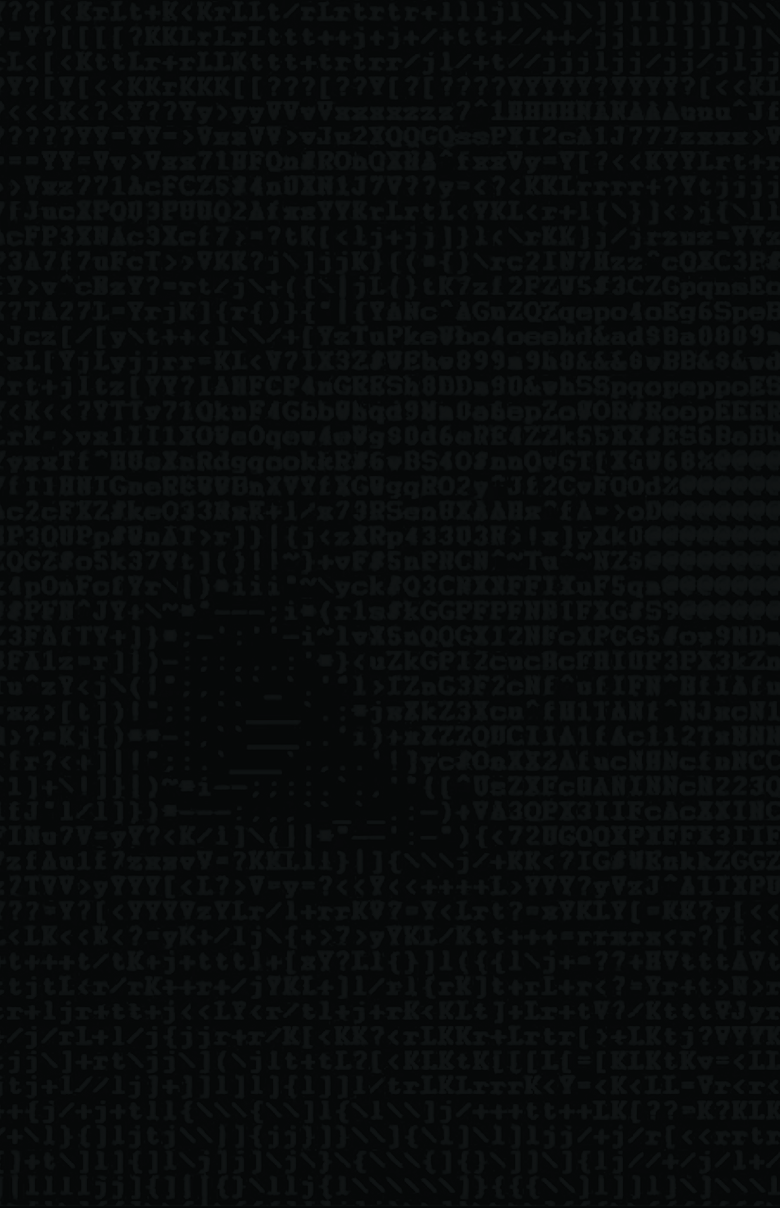
Information is
opportunity.
Opportunity
is infinite.
Everything is
information.
Information is
opportunity.
Opportunity
is infinite.
Change isn't
meant to be feared.
Change is constant.
Change fuels innovation.
Change empowers people.
Change is progress.
Change isn't
meant to be feared.
Change is constant.
Change fuels innovation.
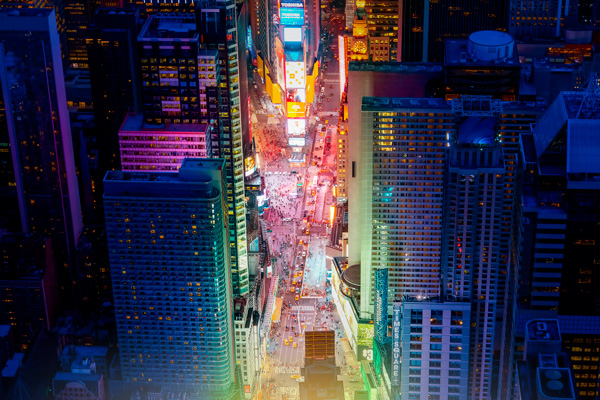
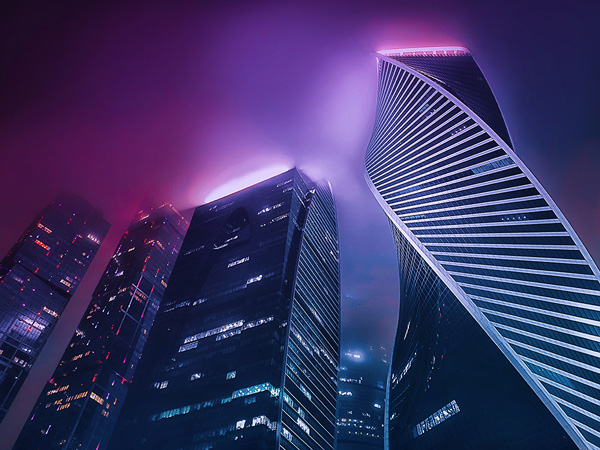
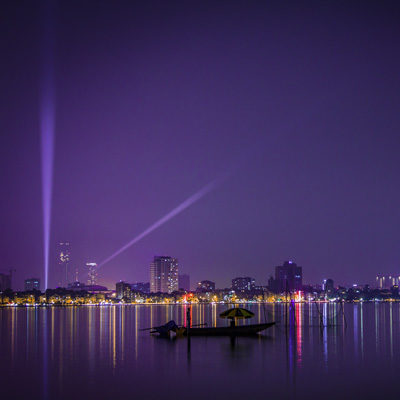
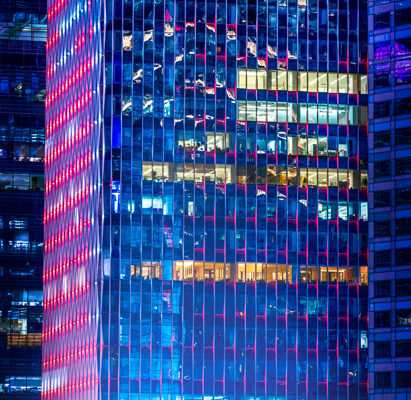
Quantity
is quality.
Talent
Ideas
Solutions
Quantity
is quality.
Talent
Ideas
Solutions
Talent is global,
opportunity is not.
प्रतिभा दुनिया भर में पाई जा सकती है, लेकिन मौके नहीं।
A tehetség globális, a lehetőség nem.
인재는 많지만 기회는 적습니다.
Nhân tài vô hạn, cơ hội hữu hạn.
人才遍布全球,机遇一缘难求
1,000+
employees
26
global offices
Beyond asset management
Our Founder, Chairman and CEO Igor Tulchinsky has applied his beliefs to projects and entities outside of WorldQuant — leveraging data, talent and prediction to tackle complex challenges beyond financial markets.
These entities, founded by Igor, operate independently from WorldQuant’s global quantitative asset management business.